

Based on this, we can construct confidence intervals
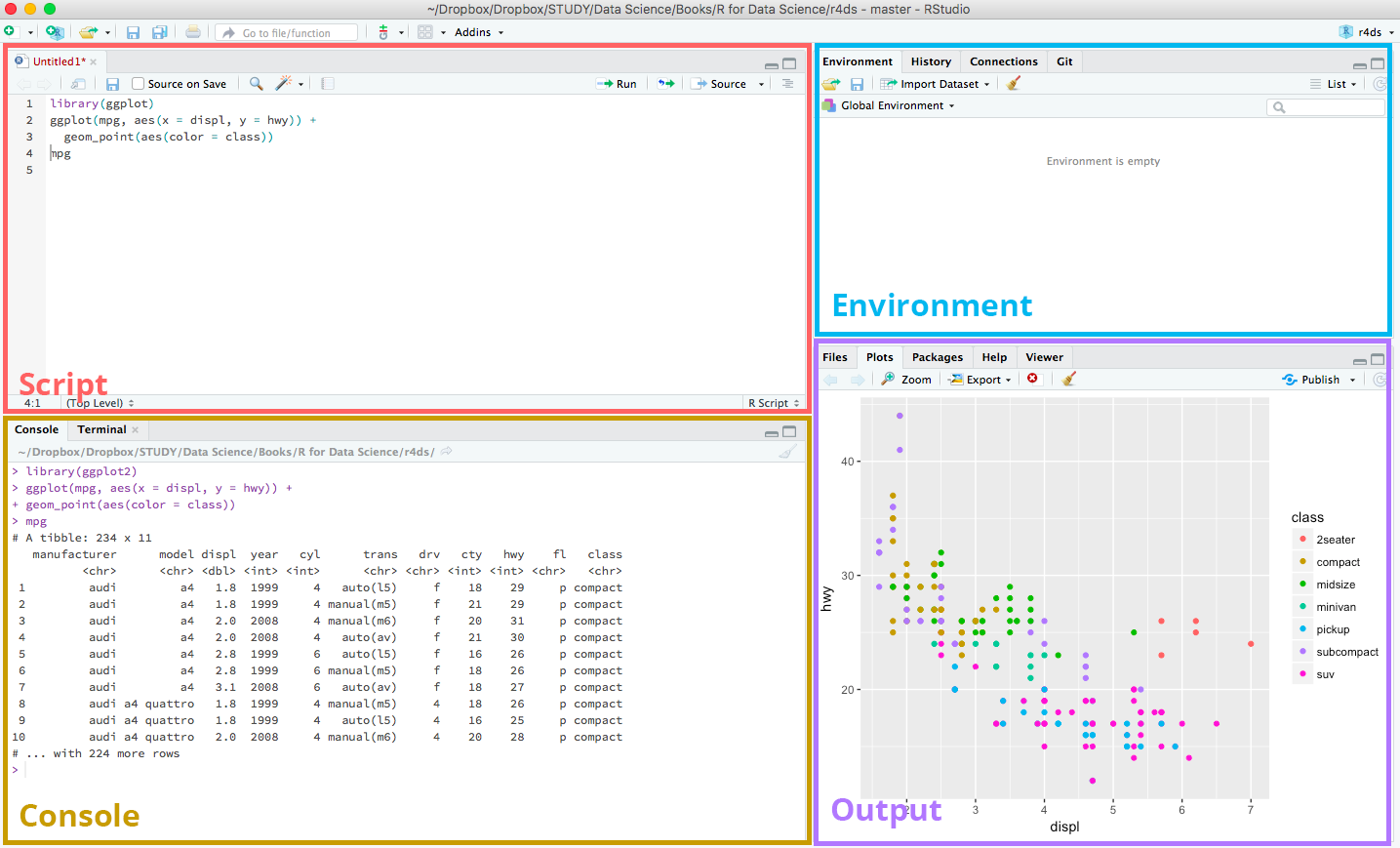
That is, assuming all model assumptions are satisfied, we can say that with 95% confidence (which is not probability) the true parameter lies in.
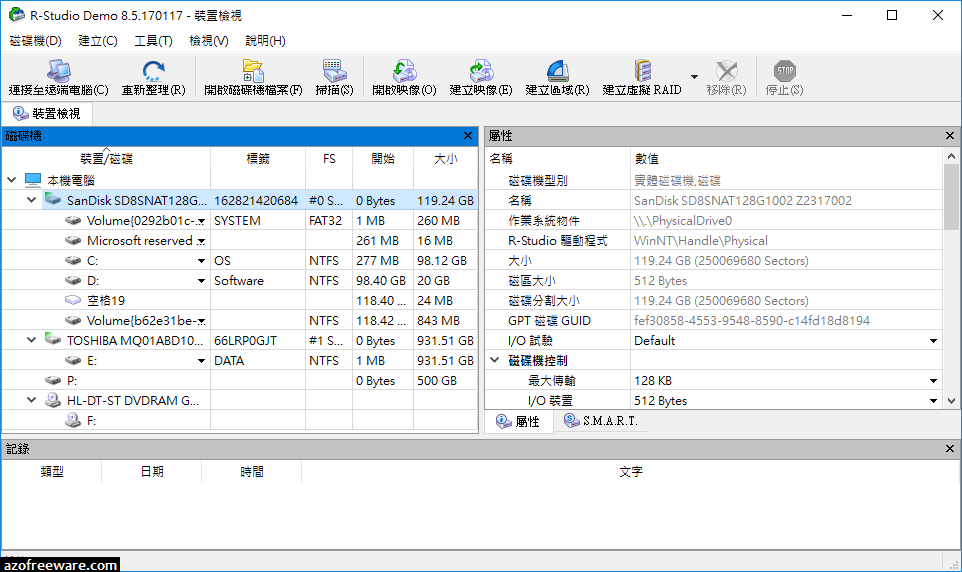
you have enough data/samples to invoke the central limit theorem, as you need to be approximately Gaussian.Note that this requires two things for this confidence interval to be valid: If is the standard error and is the estimated coefficient for feature, then a 95% confidence interval is given by. The standard error is the standard error of our estimate, which allows us to construct marginal confidence intervals for the estimate of that particular feature. For the other features, the estimates give us the expected change in the response due to a unit change in the feature. Now, when features are at their mean values, the expected response is the intercept. Note that for an arguably better interpretation, you should consider centering your features. The intercept tells us that when all the features are at, the expected response is the intercept. This includes their estimates, standard errors, t statistics, and p-values. The second thing printed by the linear regression summary call is information about the coefficients. All of this is good as it suggests correct model specification. Further, the and percentile look approximately the same distance from, and the non-outlier min and max also look about the same distance from. We can investigate this further with a boxplot of the residuals.īoxplot(model],main='Boxplot: Residuals',ylab='residual value') However, in this case, not holding may indicate an outlier rather than a symmetry violation. The max and min should also have similar magnitude. They would be equal under a symmetric mean distribution. Further, the 3Q and 1Q should be close to each other in magnitude. The median should be close to as the mean of the residuals is, and symmetric distributions have median=mean. The residual summary statistics give information about the symmetry of the residual distribution. As a consequence the residuals should as well. One of the assumptions for hypothesis testing is that the errors follow a Gaussian distribution. The first info printed by the linear regression summary after the formula is the residual summary statistics. Residual standard error: 0.2158 on 501 degrees of freedom
